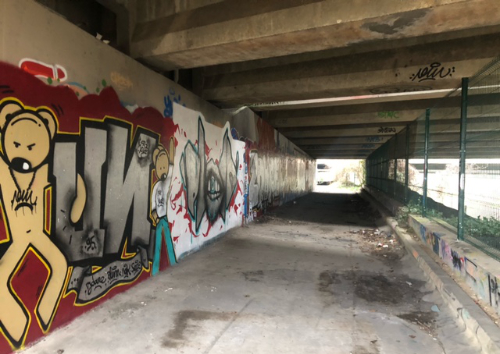
DL-SSOA
Deep Learning applied to structural monitoring of engineering structures
Scientific responsibility :
- Franziska Schmidt
- Archour Debiane
Methodological axes :
Funding :
- Région Île-de-France
- CERTIA
Summary :
In France, 10% of the value of the country’s road network comes from engineering structures (estimated at between 200,000 and 250,000 bridges). The road network carries 88% of passenger and freight traffic and therefore plays a vital role in the country’s communications and economy. However, a survey by the IPSOS institute revealed a rise in dissatisfaction among the French, from 17% to 22% in two years. This follows the collapse of the Morandi bridge in Genoa in 2018, which revived the debate on the safety of engineering structures and the memory of the Mont Blanc tunnel disaster in 1999. This feeling is justified when we know that, according to the Ministry of Ecological Transition and Solidarity in 2018, a third of the bridges managed by the state are in need of repair, and that not all metal bridges are in good condition. The fact that France’s road infrastructure is ageing is perfectly normal, given that the theoretical life of a civil engineering structure is estimated at 100 years. However, a large number of bridges were built before the year 2000 and the introduction of European standards, which means that their lifespan is less than the expected 100 years and tends to be closer to 70 years. It is difficult to manage these assets because the only way to monitor deterioration is visually, which is far from sufficient to detect certain pathologies, such as corrosion, that develop inside the materials. The problem must therefore be tackled upstream. This thesis is based on the premise that by recording and analysing the behaviour of the structure over a sufficiently long period (learning period), it will be possible to obtain a dictionary of signals compatible with the state of deterioration of the structure and its load, and to apply deep learning methods to detect changes in this behaviour.
PhD student : Mohamed Abdelillah Fidma